Matlab Tutorial - M Files (Scripts)
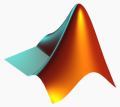
M-files are macros of MATLAB commands. The M-files are stored as ordinary text files with the extension m, filename.m. An M-file can be either a function with input and output variables or a list of commands.
Where should we put the M-files?
MATLAB requires that the M-file must be stored either in the working directory or in a directory that is specified in the MATLAB path list.
On Windows, the default location is C:\Documents and Settings\user\My Documents\MATLAB. We can check it by New->Script and then Save As:
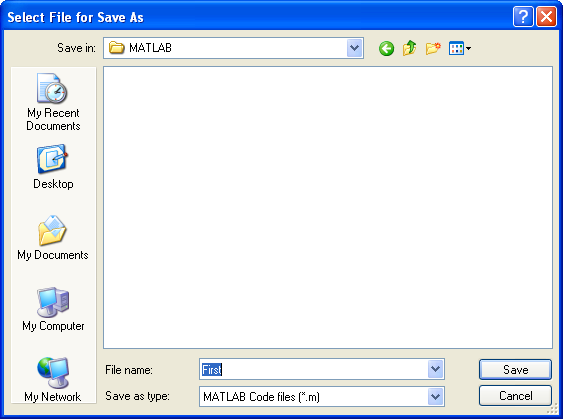
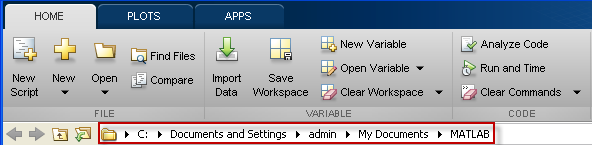
Suppose we want to have the M-files in C:\Documents and Settings\user\My Documents\MATLAB\FFT. We can let Matlab know where it can find the file in two ways:
- Change the working directory by issuing cd path command:
>> pwd ans = C:\Documents and Settings\admin\My Documents\MATLAB >> cd FFT >> pwd ans = C:\Documents and Settings\admin\My Documents\MATLAB\FFT
- Add the directory to the path.
- Permanent addition to the path : edit the ....\MATLAB\matlabrc.m file
- Temporary modification to the path : issue a command, path(path,'C:\Documents and Settings\user\My Documents\MATLAB\FFT') withing Matlab
In this section, we'll see how the M-files are used.
Let's create a file in our working directory (the default directory mentioned in previous section). Name it as cexp.m that has the following commands:
function fval = cexp(a,b) fval = exp(a+b*i)
Then within the command window:
>> x = 1; >> y = 2; >> cexp(x,y) fval = -1.1312 + 2.4717i ans = -1.1312 + 2.4717i >>
Ph.D. / Golden Gate Ave, San Francisco / Seoul National Univ / Carnegie Mellon / UC Berkeley / DevOps / Deep Learning / Visualization