scikit-learn : Data Compression via Dimensionality Reduction III - Nonlinear mappings via kernel principal component (KPCA) analysis
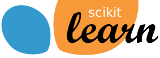
In real-world applications, linear transformation such as PCA and LDA are not the best technique for dimensionality reduction.
In this section, a kernel PCA will be introduced. It is conceptually similar to kernel SVM. Using kernel PCA, we will see how to transform data that is not linearly separable onto a lower-dimensional subspace suitable for linear classifiers.
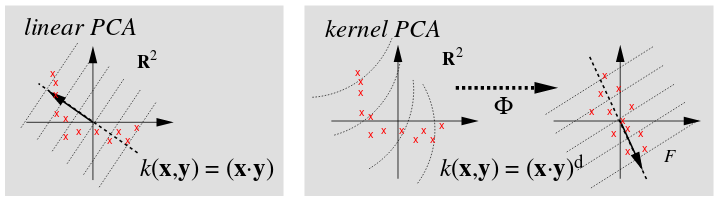
picture source : Scholkopf B, Smola A, and Muller KR, Kernel principal component analysis, 1999
KPCA is using a kernel trick with standard PCA. The resulting algorithm is similar, except that every dot product is replaced by a nonlinear kernel function.
The kernel is related to the transform $\phi (\mathbf x)$, and the resulting $n \times n$ kernel matrix looks like this:
$$ \mathbf K = \mathbf k(\mathbf x, \mathbf y) = (\phi (\mathbf x), \phi (\mathbf y)) = \phi (\mathbf x)^T \phi (\mathbf y)$$The algorithm collects the top $k$ eigenvectors of the centered kernel matrix based on their corresponding eigenvalues, which are ranked by decreasing magnitude. But unlike standard PCA, the eigenvectors of KPCA are not the principal component axes but the samples projected onto those axes.
As we can see from the left picture, the two half-moon shapes are not linearly separable. The plot on the right side is how the dataset looks like if we project it onto the principal components via standard PCA:
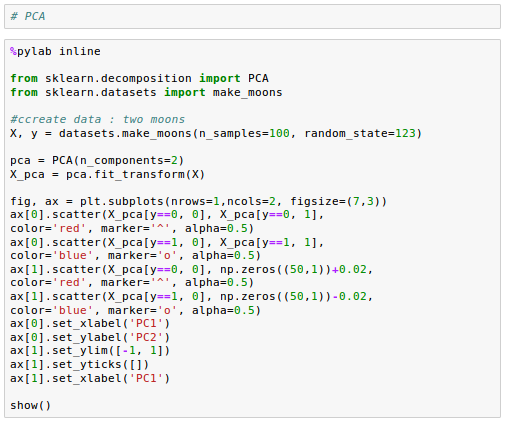
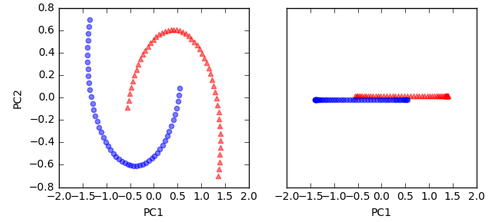
As expected, the linear standard PCA classifier was unable to separate the dataset. The plot used the first principal component only, and the triangular samples slightly shifted upwards and the circular samples slightly downwards to demonstrate the overlap.
So, in the next section, we want to try Kernel PCA rather than standard PCA.
We are going to implement an RBF kernel PCA of Scikit-learn:
Kernel PCA - moon dataset:
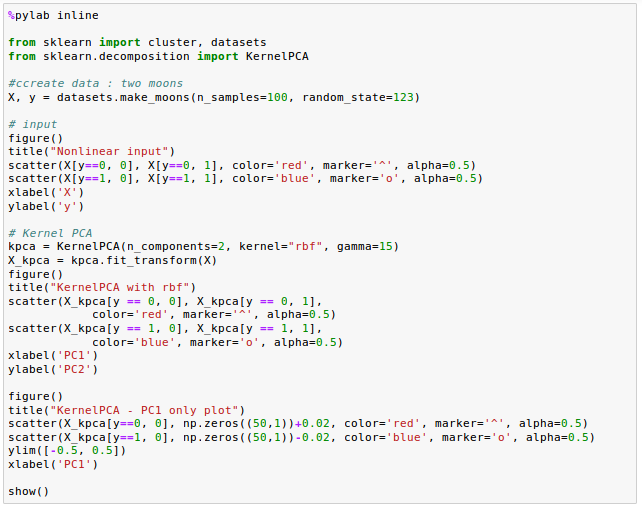
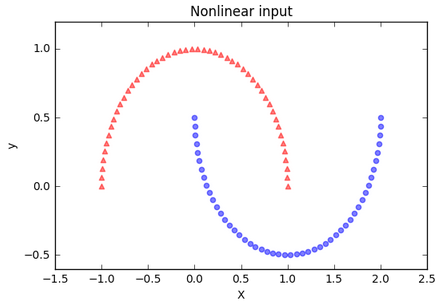
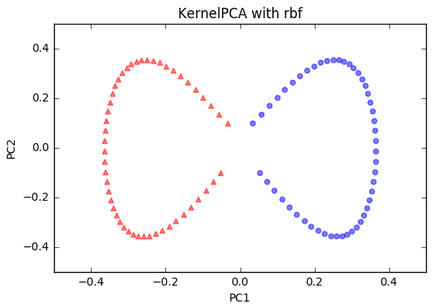
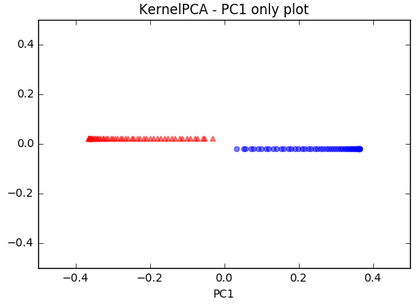
Kernel PCA - circle dataset. This is a nonlinear problem with concentric circles:
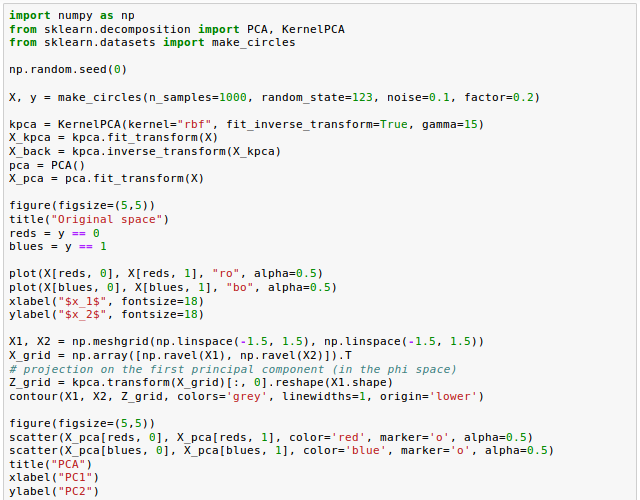
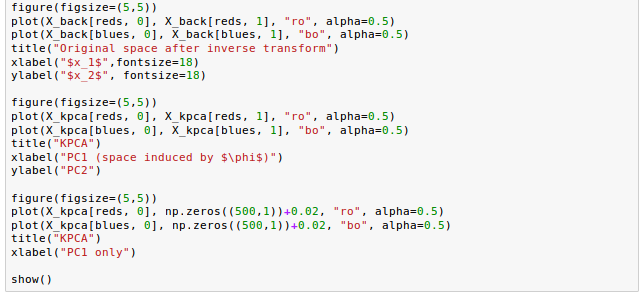
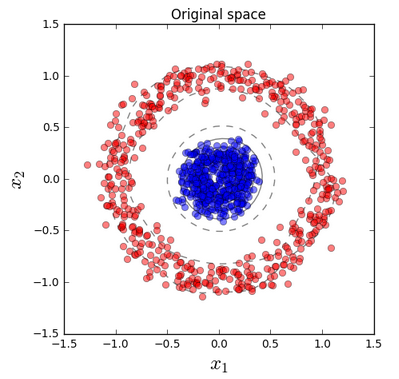
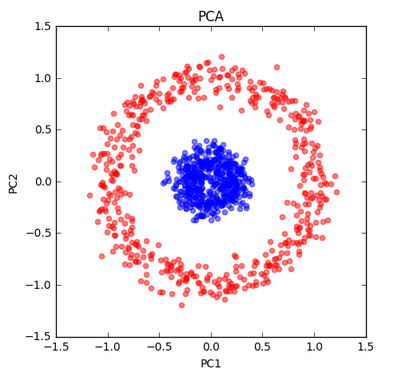
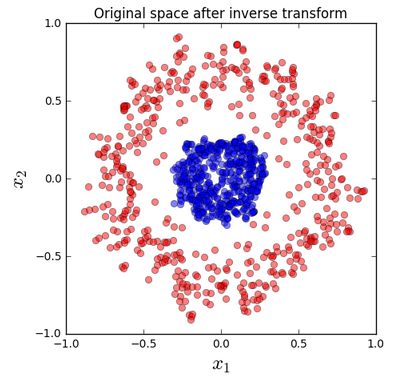
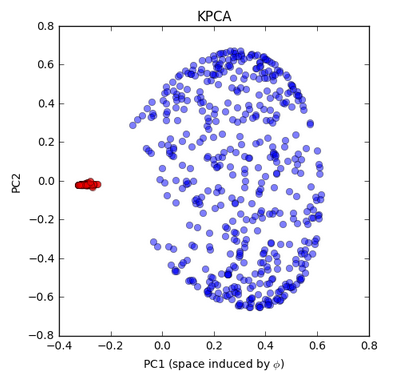
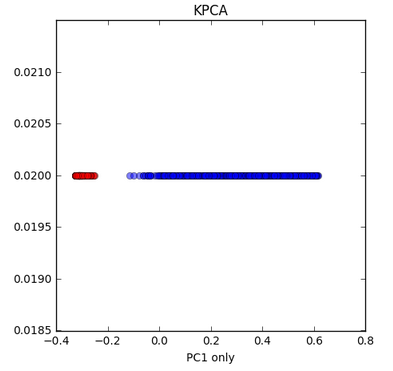
Jupyter notebook is available from Github:
Machine Learning with scikit-learn
scikit-learn installation
scikit-learn : Features and feature extraction - iris dataset
scikit-learn : Machine Learning Quick Preview
scikit-learn : Data Preprocessing I - Missing / Categorical data
scikit-learn : Data Preprocessing II - Partitioning a dataset / Feature scaling / Feature Selection / Regularization
scikit-learn : Data Preprocessing III - Dimensionality reduction vis Sequential feature selection / Assessing feature importance via random forests
Data Compression via Dimensionality Reduction I - Principal component analysis (PCA)
scikit-learn : Data Compression via Dimensionality Reduction II - Linear Discriminant Analysis (LDA)
scikit-learn : Data Compression via Dimensionality Reduction III - Nonlinear mappings via kernel principal component (KPCA) analysis
scikit-learn : Logistic Regression, Overfitting & regularization
scikit-learn : Supervised Learning & Unsupervised Learning - e.g. Unsupervised PCA dimensionality reduction with iris dataset
scikit-learn : Unsupervised_Learning - KMeans clustering with iris dataset
scikit-learn : Linearly Separable Data - Linear Model & (Gaussian) radial basis function kernel (RBF kernel)
scikit-learn : Decision Tree Learning I - Entropy, Gini, and Information Gain
scikit-learn : Decision Tree Learning II - Constructing the Decision Tree
scikit-learn : Random Decision Forests Classification
scikit-learn : Support Vector Machines (SVM)
scikit-learn : Support Vector Machines (SVM) II
Flask with Embedded Machine Learning I : Serializing with pickle and DB setup
Flask with Embedded Machine Learning II : Basic Flask App
Flask with Embedded Machine Learning III : Embedding Classifier
Flask with Embedded Machine Learning IV : Deploy
Flask with Embedded Machine Learning V : Updating the classifier
scikit-learn : Sample of a spam comment filter using SVM - classifying a good one or a bad one
Machine learning algorithms and concepts
Batch gradient descent algorithmSingle Layer Neural Network - Perceptron model on the Iris dataset using Heaviside step activation function
Batch gradient descent versus stochastic gradient descent
Single Layer Neural Network - Adaptive Linear Neuron using linear (identity) activation function with batch gradient descent method
Single Layer Neural Network : Adaptive Linear Neuron using linear (identity) activation function with stochastic gradient descent (SGD)
Logistic Regression
VC (Vapnik-Chervonenkis) Dimension and Shatter
Bias-variance tradeoff
Maximum Likelihood Estimation (MLE)
Neural Networks with backpropagation for XOR using one hidden layer
minHash
tf-idf weight
Natural Language Processing (NLP): Sentiment Analysis I (IMDb & bag-of-words)
Natural Language Processing (NLP): Sentiment Analysis II (tokenization, stemming, and stop words)
Natural Language Processing (NLP): Sentiment Analysis III (training & cross validation)
Natural Language Processing (NLP): Sentiment Analysis IV (out-of-core)
Locality-Sensitive Hashing (LSH) using Cosine Distance (Cosine Similarity)
Artificial Neural Networks (ANN)
[Note] Sources are available at Github - Jupyter notebook files1. Introduction
2. Forward Propagation
3. Gradient Descent
4. Backpropagation of Errors
5. Checking gradient
6. Training via BFGS
7. Overfitting & Regularization
8. Deep Learning I : Image Recognition (Image uploading)
9. Deep Learning II : Image Recognition (Image classification)
10 - Deep Learning III : Deep Learning III : Theano, TensorFlow, and Keras
Ph.D. / Golden Gate Ave, San Francisco / Seoul National Univ / Carnegie Mellon / UC Berkeley / DevOps / Deep Learning / Visualization